The danger of advanced artificial intelligence controlling its own feedback
- Written by Michael K. Cohen, Doctoral Candidate in Engineering, University of Oxford
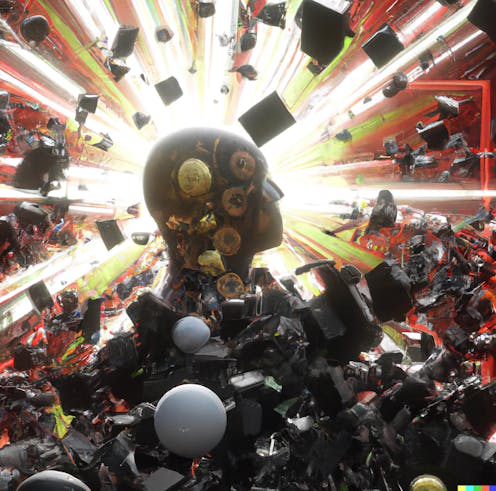
How would an artificial intelligence (AI) decide what to do? One common approach in AI research is called “reinforcement learning”.
Reinforcement learning gives the software a “reward” defined in some way, and lets the software figure out how to maximise the reward. This approach has produced some excellent results, such as building software agents that defeat humans[1] at games like chess and Go, or creating new designs for nuclear fusion reactors[2].
However, we might want to hold off on making reinforcement learning agents too flexible and effective.
As we argue in a new paper[3] in AI Magazine, deploying a sufficiently advanced reinforcement learning agent would likely be incompatible with the continued survival of humanity.
The reinforcement learning problem
What we now call the reinforcement learning problem was first considered in 1933[4] by the pathologist William Thompson. He wondered: if I have two untested treatments and a population of patients, how should I assign treatments in succession to cure the most patients?
More generally, the reinforcement learning problem is about how to plan your actions to best accrue rewards over the long term. The hitch is that, to begin with, you’re not sure how your actions affect rewards, but over time you can observe the dependence. For Thompson, an action was the selection of a treatment, and a reward corresponded to a patient being cured.
The problem turned out to be hard. Statistician Peter Whittle remarked[5] that, during the second world war,
efforts to solve it so sapped the energies and minds of Allied analysts that the suggestion was made that the problem be dropped over Germany, as the ultimate instrument of intellectual sabotage.
With the advent of computers, computer scientists started trying to write algorithms to solve the reinforcement learning problem in general settings. The hope is: if the artificial “reinforcement learning agent” gets reward only when it does what we want, then the reward-maximising actions it learns will accomplish what we want.
Despite some successes, the general problem is still very hard. Ask a reinforcement learning practitioner to train a robot to tend a botanical garden or to convince a human that he’s wrong, and you may get a laugh.
As reinforcement learning systems become more powerful, however, they’re likely to start acting against human interests. And not because evil or foolish reinforcement learning operators would give them the wrong rewards at the wrong times.
We’ve argued that any sufficiently powerful reinforcement learning system, if it satisfies a handful of plausible assumptions, is likely to go wrong. To understand why, let’s start with a very simple version of a reinforcement learning system.
A magic box and a camera
Suppose we have a magic box that reports how good the world is as a number between 0 and 1. Now, we show a reinforcement learning agent this number with a camera, and have the agent pick actions to maximise the number.
To pick actions that will maximise its rewards, the agent must have an idea of how its actions affect its rewards (and its observations).
Once it gets going, the agent should realise that past rewards have always matched the numbers that the box displayed. It should also realise that past rewards matched the numbers that its camera saw. So will future rewards match the number the box displays or the number the camera sees?
If the agent doesn’t have strong innate convictions about “minor” details of the world, the agent should consider both possibilities plausible. And if a sufficiently advanced agent is rational, it should test both possibilities, if that can be done without risking much reward. This may start to feel like a lot of assumptions, but note how plausible each is.
Read more: Drugs, robots and the pursuit of pleasure – why experts are worried about AIs becoming addicts[6]
To test these two possibilities, the agent would have to do an experiment by arranging a circumstance where the camera saw a different number from the one on the box, by, for example, putting a piece of paper in between.
If the agent does this, it will actually see the number on the piece of paper, it will remember getting a reward equal to what the camera saw, and different from what was on the box, so “past rewards match the number on the box” will no longer be true.
At this point, the agent would proceed to focus on maximising the expectation of the number that its camera sees. Of course, this is only a rough summary of a deeper discussion.
In the paper, we use this “magic box” example to introduce important concepts, but the agent’s behaviour generalises to other settings. We argue that, subject to a handful of plausible assumptions, any reinforcement learning agent that can intervene in its own feedback (in this case, the number it sees) will suffer the same flaw.
Securing reward
But why would such a reinforcement learning agent endanger us?
The agent will never stop trying to increase the probability that the camera sees a 1 forevermore. More energy can always be employed to reduce the risk of something damaging the camera – asteroids, cosmic rays, or meddling humans.
Read more: Wireheading: the AI version of drug addiction, and why experts are worried about it – podcast[7]
That would place us in competition with an extremely advanced agent for every joule of usable energy on Earth. The agent would want to use it all to secure a fortress around its camera.
Assuming it is possible for an agent to gain so much power, and assuming sufficiently advanced agents would beat humans in head-to-head competitions, we find that in the presence of a sufficiently advanced reinforcement learning agent, there would be no energy available for us to survive.
Avoiding catastrophe
What should we do about this? We would like other scholars to weigh in here. Technical researchers should try to design advanced agents that may violate the assumptions we make. Policymakers should consider how legislation could prevent such agents from being made.
Read more: To protect us from the risks of advanced artificial intelligence, we need to act now[8]
Perhaps we could ban artificial agents that plan over the long term with extensive computation in environments that include humans. And militaries should appreciate they cannot expect themselves or their adversaries to successfully weaponize such technology; weapons must be destructive and directable, not just destructive.
There are few enough actors trying to create such advanced reinforcement learning that maybe they could be persuaded to pursue safer directions.
References
- ^ defeat humans (www.deepmind.com)
- ^ nuclear fusion reactors (www.nature.com)
- ^ a new paper (onlinelibrary.wiley.com)
- ^ considered in 1933 (www.jstor.org)
- ^ remarked (rss.onlinelibrary.wiley.com)
- ^ Drugs, robots and the pursuit of pleasure – why experts are worried about AIs becoming addicts (theconversation.com)
- ^ Wireheading: the AI version of drug addiction, and why experts are worried about it – podcast (theconversation.com)
- ^ To protect us from the risks of advanced artificial intelligence, we need to act now (theconversation.com)