50,000 Australians a day are being tested for COVID-19. How to solve the maths that says the number should be 6.5 million
- Written by Richard Holden, Professor of Economics, UNSW
As Victoria grapples with a second-wave outbreak of COVID-19, the importance of large-scale testing has again been highlighted.
Without its “testing blitz[1]” aiming at 10,000 tests a day, the extent of the outbreak would have been invisible for much longer.
Read more: Australia's coronavirus testing rates are some of the best in the world – compare our stats using this interactive[2]
Australia-wide, we’ve so far achieved a seven-day rolling average of a little more than 50,000 tests a day[3]. The highest single-day record was 66,525 on June 22.
Since the beginning of the pandemic, proponents of mass testing having been arguing the need to test dramatically larger numbers.
One is US economist Paul Romer, who shared the 2018 Nobel Prize for economics[4] for his work on the importance of knowledge and ideas in the economy. In late March he modelled[5] a “frequent testing policy” in which:
7% of the population is randomly selected for testing each day. Over the 500 days illustrated in the plots and the animation, this means that the average person is tested about 30 times in 500 days – roughly once very two weeks.
This rate of testing would mean testing close to 2 million Australians a day. But even that is probably not enough.
The mathematics of mass testing
To appreciate why an even large number of tests is needed, imagine purely random testing is being used. That is, testing not focused on “hot spots” as has been the case in Melbourne.
Testing, tracing and isolating will only be effective if, on average, the process identifies cases before those people transmit the virus. Epidemiologists call the number of infections caused by a single case the “reproduction” (R) number. If it’s less than 1, the pandemic will die out. If it’s more than 1, the virus spreads.
When uncontrolled, COVID-19 spreads roughly once every six days[6]. This corresponds to a reproduction number of 2.5 over the 15 days a person with COVID-19 is generally infectious.
Moreover, as Romer notes[7], current tests are far from perfect. He assumes a 20% false negative rate (tests results saying someone doesn’t have COVID-19 when they do) and a 1% false positive rate (results saying someone has it when they don’t).
The mathematics says to control the disease we need to test the entire population roughly every four days.
In a paper published by Harvard University’s Edmond J. Safra Center for Ethics[8], Divya Siddarth and Glen Weyl] calculate the 20% who are false negatives will, on average, pass the virus to 2.5 people. The other 80% of cases are caught on average halfway through the testing cycle.
To put it in basic maths terms, if we test people every “x” days, we catch those infections on average after half that time (x/2 days). To keep the effective reproduction rate at, say, 0.75 we need “x” to be 3.75. That means testing everyone roughly once every four days.
And that would mean testing more than 6.5 million Australians a day. Yikes!
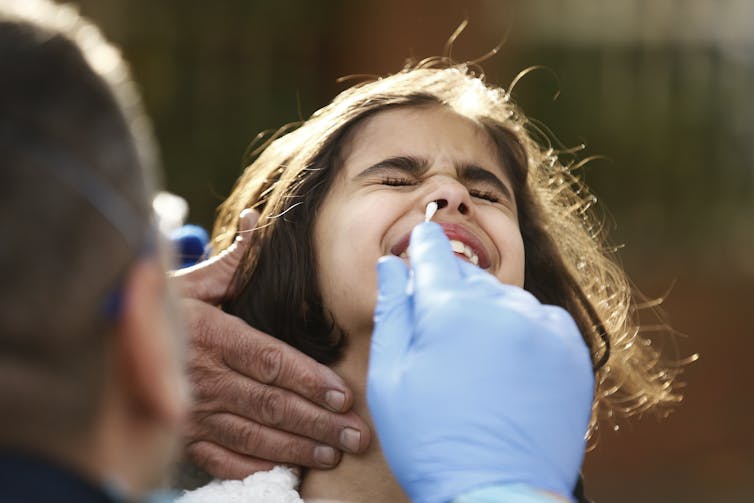
Asymptomatic cases
Given the scale needed to make random testing a success, it is perhaps not surprising authorities have opted for targeted testing – focusing on transmission hot spots.
But Siddarth and Weyl explain the fatal flaw with any testing strategy reacting only to symptomatic cases:
By the time symptomatic patients show up, they will already have infected .833 people. Furthermore, 20% of those infected will be asymptomatic throughout the time they have the disease, and 20% of those tested will yield false negatives. This means that a policy of only testing those who present with symptoms and only quarantining those who test positive will lead an average infected individual to infect others at a rate of 1.4.
Being above 1, this means the virus still grows exponentially.
Read more: Coronavirus: asymptomatic people can still develop lung damage[9]
Testing with contact tracing
A better solution is to test based on rigorous contact tracing of known infections. This is why governments pinned such great hopes on the technology of tracing apps such as Australia’s COVIDSafe.
Siddarth and Weyl consider a kind of best-case scenario that traces everyone an infectious person has come into contact with, and everyone those people have come into contact with as well. They calculate this could lead to tracking down 75% of cases. Other transmission would be pursued through prompt testing of everyone with symptoms.
In the US this would require about 2 million tests a day. In Australia it would need about 150,000 tests a day – three times as many tests as are being done now.
Group testing
An intriguing solution is “group testing”. This idea has been around since the 1940s and involves pooling patient specimens for testing. If the pooled test is negative, the whole group is cleared. If the test is positive, more focused testing is done to identify individual cases.
Read more: Group testing for coronavirus – called pooled testing – could be the fastest and cheapest way to increase screening nationwide[10]
The question, then, is what is the optimal group-testing strategy? For instance, what is the best group size to choose? Should some people be placed in multiple groups? Should there be multiple stages of group testing?
In a US National Bureau of Economic Research working paper[11] published this month, four scholars from the University of California Berkeley show how machine learning can help to determine the optimal strategy.
For example, optimal group test size depends on the prevalence of the virus in the population. By estimating individual risk profiles – a person’s age, pre-existing health problems, where they live, if they work in a job that exposes them to risks, and so on – it is possible to target tests more efficiently than if treating everyone as equally at risk.
The goal is to improve predictive accuracy by incorporating as many observable characteristics that may influence risk as possible. This is a classic problem for “supervised machine learning”. Using machine learning could make group testing perhaps four to five times more efficient, the Berkeley researchers suggest.
Done this way, we might be able to achieve an effective strategy by testing as few as 30,000 to 40,000 Australians a day.
But the approach will need to be very different from now.
Our pre-vaccine future
Until an effective vaccine is found and widely deployed, testing is crucial to control COVID-19.
As the Berkeley authors emphasise, modern analytic techniques can make “high-frequency, intelligent group testing a powerful new tool in the fight against COVID-19, and potentially other infectious diseases”.
We need all the tools we can find.
References
- ^ testing blitz (www.abc.net.au)
- ^ Australia's coronavirus testing rates are some of the best in the world – compare our stats using this interactive (theconversation.com)
- ^ 50,000 tests a day (www.covid19data.com.au)
- ^ 2018 Nobel Prize for economics (www.nobelprize.org)
- ^ he modelled (paulromer.net)
- ^ once every six days (wwwnc.cdc.gov)
- ^ as Romer notes (paulromer.net)
- ^ Edmond J. Safra Center for Ethics (ethics.harvard.edu)
- ^ Coronavirus: asymptomatic people can still develop lung damage (theconversation.com)
- ^ Group testing for coronavirus – called pooled testing – could be the fastest and cheapest way to increase screening nationwide (theconversation.com)
- ^ working paper (www.nber.org)
Authors: Richard Holden, Professor of Economics, UNSW