AI weather models can now beat the best traditional forecasts
- Written by Vassili Kitsios, Senior Research Scientist, Climate Forecasting, CSIRO
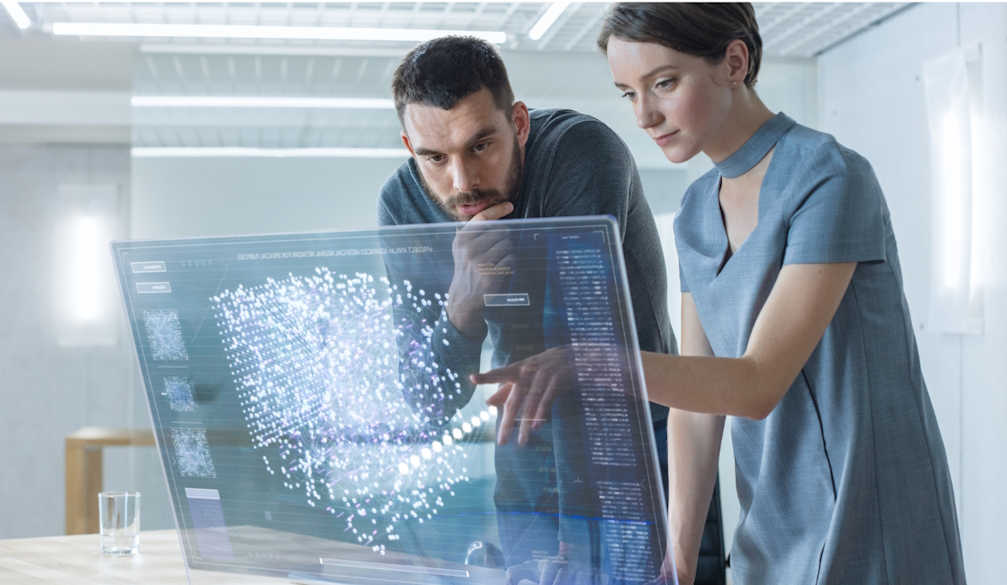
A new machine-learning weather prediction model called GenCast can outperform the best traditional forecasting systems in at least some situations, according to a paper by Google DeepMind researchers[1] published today in Nature.
Using a diffusion model approach similar to artificial intelligence (AI) image generators, the system generates multiple forecasts to capture the complex behaviour of the atmosphere. It does so with a fraction of the time and computing resources required for traditional approaches.
How weather forecasts work
The weather predictions we use in practice are produced by running multiple numerical simulations of the atmosphere.
Each simulation starts from a slightly different estimate of the current weather. This is because we don’t know exactly what the weather is at this instant everywhere in the world. To know that, we would need sensor measurements everywhere.
These numerical simulations use a model of the world’s atmosphere divided into a grid of three-dimensional blocks. By solving equations describing the fundamental physical laws of nature, the simulations predict what will happen in the atmosphere.
Known as general circulation models, these simulations need a lot of computing power. They are usually run at high-performance supercomputing facilities.
Machine-learning the weather
The past few years have seen an explosion in efforts to produce weather prediction models using machine learning[2]. Typically, these approaches don’t incorporate our knowledge of the laws of nature the way general circulation models do.
Most of these models use some form of neural network to learn patterns in historical data and produce a single future forecast. However, this approach produces predictions that lose detail as they progress into the future, gradually becoming “smoother”. This smoothness is not what we see in real weather systems.
Researchers at Google’s DeepMind AI research lab have just published a paper in Nature[3] describing their latest machine-learning model, GenCast.
GenCast mitigates this smoothing effect by generating an ensemble of multiple forecasts. Each individual forecast is less smooth, and better resembles the complexity observed in nature.
The best estimate of the actual future then comes from averaging the different forecasts. The size of the differences between the individual forecasts indicates how much uncertainty there is.
According to the GenCast paper, this probabilistic approach creates more accurate forecasts than the best numerical weather prediction system in the world – the one at the European Centre for Medium-Range Weather Forecasts[4].
Generative AI – for weather
GenCast is trained on what is called reanalysis data from the years 1979 to 2018. This data is produced by the kind of general circulation models we talked about earlier, which are additionally corrected to resemble actual historical weather observations to produce a more consistent picture of the world’s weather.
The GenCast model makes predictions of several variables such as temperature, pressure, humidity and wind speed at the surface and at 13 different heights, on a grid that divides the world up into 0.25-degree regions of latitude and longitude.
GenCast is what is called a “diffusion model”, similar to AI image generators. However, instead of taking text and producing an image, it takes the current state of the atmosphere and produces an estimate of what it will be like in 12 hours.
This works by first setting the values of the atmospheric variables 12 hours into the future as random noise. GenCast then uses a neural network to find structures in the noise that are compatible with the current and previous weather variables. An ensemble of multiple forecasts can be generated by starting with different random noise.
Forecasts are run out to 15 days, taking 8 minutes on a single processor called a tensor processor unit (TPU). This is significantly faster than a general circulation model. The training of the model took five days using 32 TPUs.
Machine-learning forecasts could become more widespread in the coming years as they become more efficient and reliable.
However, classical numerical weather prediction and reanalysed data will still be required. Not only are they needed to provide the initial conditions for the machine learning weather forecasts, they also produce the input data to continually fine-tune the machine learning models.
What about the climate?
Current machine learning weather forecasting systems are not appropriate for climate projections, for three reasons.
Firstly, to make weather predictions weeks into the future, you can assume that the ocean, land and sea ice won’t change. This is not the case for climate predictions over multiple decades.
Secondly, weather prediction is highly dependent on the details of the current weather. However, climate projections are concerned with the statistics of the climate decades into the future, for which today’s weather is irrelevant. Future carbon emissions are the greater determinant of the future state of the climate.
Thirdly, weather prediction is a “big data” problem. There are vast amounts of relevant observational data, which is what you need to train a complex machine learning model.
Climate projection is a “small data” problem, with relatively little available data. This is because the relevant physical phenomena (such as sea levels or climate drivers such as the El Niño–Southern Oscillation) evolve much more slowly than the weather.
There are ways to address these problems. One approach is to use our knowledge of physics to simplify our models[5], meaning they require less data for machine learning.
Another approach is to use physics-informed neural networks[6] to try to fit the data and also satisfy the laws of nature. A third is to use physics to set “ground rules”[7] for a system, then use machine learning to determine the specific model parameters.
Machine learning has a role to play in the future of both weather forecasting and climate projections. However, fundamental physics – fluid mechanics[8] and thermodynamics – will continue to play a crucial role.
References
- ^ a paper by Google DeepMind researchers (doi.org)
- ^ using machine learning (github.com)
- ^ a paper in Nature (doi.org)
- ^ European Centre for Medium-Range Weather Forecasts (www.ecmwf.int)
- ^ simplify our models (doi.org)
- ^ physics-informed neural networks (doi.org)
- ^ use physics to set “ground rules” (doi.org)
- ^ fluid mechanics (ww.afms.org.au)
Read more https://theconversation.com/ai-weather-models-can-now-beat-the-best-traditional-forecasts-245168