The A/B Testing Revolution: How AI Optimized Landing Pages Without Human Input
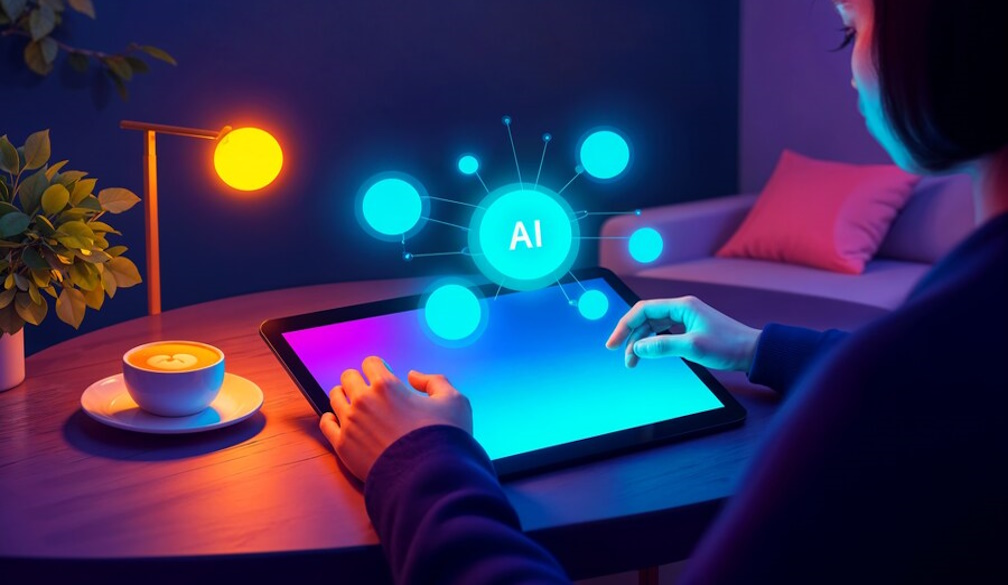
A/B testing was always integral to the web-based marketing world. Was there a button that converted better? Marketing could pit one against the other and see which option worked better. This was always through human observation, and over time, as days or weeks would pass before enough data was collected and processed. But with the ability to put AI in place, A/B testing can become more automated, more rapid, and more intelligent. Buttons know when to switch over time on their own without a need for human intervention. Therefore, testing is simpler and more effective.
From Manual Testing to AI-Driven Optimization
A/B testing, for instance, means that marketers create different versions of a landing page to test against one another, and they manually review the results to determine which was more effective. This is time-consuming and energy-expanding for just one test imagine thousands of pages or multiple variables. But that's unnecessary with the advent of AI. The testing and adjustments occur via AI integration without human-driven page creation or input. Essentially, an AI platform can create different versions of a landing page to test headlines, images, calls to action, etc., based on AI-collected data about a specific audience. Anybiz.io helps facilitate this by using intelligent automation to streamline these testing processes and optimize results in real-time.
Furthermore, AI can adjust in real time based on feedback and actions taken by users on the page. That means that a visitor will see one rendering of a landing page over time compared to someone who comes after them, as AI determines what's working and what's not and adjusts in the moment. No manual assessment is needed. Where A/B testing is done at a specific time in the human realm, with AI, it's an ongoing A/B testing effort to enhance user experience and conversion rates.
Automated Experimentation and Real-Time Adjustments
Maybe the best benefit of AI A/B testing is real-time experimentation automation, reducing the drawn-out processes of manual A/B testing. For instance, with manual testing, marketers may discover days or weeks later that not enough data was collected to determine which variation was more effective. Such delayed discovery wastes not only time but money by then, adjustments could have already been made. But AI reduces this time, as software can successfully test multiple variations of any one landing page all at once and alter as needed based on consistent user feedback in that moment.
AI can automate A/B testing on landing pages at scale in time, color, words, images, positioning, etc. In real-time, AI can evaluate what changes are working best to promote conversion. For example, AI might discover that a red call-to-action button leads to more sign-ups than a blue one, or a headline that's a question prompts more response than a statement. Ultimately, what a human could manage over time with a lot of work is not to this exact micro-level of detail that AI does naturally and spontaneously.
Whereas AI processes user interaction data, it doesn't just adjust and evaluate in real time. It applies in real time, as well. For example, if one version of a landing page gets more clicks, the AI will direct all users to that page. Simultaneously, it will still adjust the other versions, trying to change them to achieve the same success as the one getting all the clicks. These real-time applications both positive and negative make for a smoother experience for users in the moment, as well as over time.
What differentiates AI A/B testing from regular A/B testing is the speed at which it all happens. Regular A/B testing can be a bit slow because it needs tons of data before it can render a viable prediction. The longer the campaign runs, the more results the marketing team can get, albeit testing on a more niche level or with a smaller audience for more targeted results. Therefore, getting an adequate sample size can take some time. But AI can assess this data in hours or minutes and provide a recommendation for marketers to implement far sooner than they would ever want or expect. Therefore, companies can have their pages optimized in almost real-time and, without AI, they would find a campaign stalled before it can even get off the ground, over and over again.
With AI, real-time optimization means that not only can such flexibility be achieved, but that it's practically assured. A/B testing, for example, does not require marketers to put in place what's needed, wait for data collection, then wait for further analysis and implementation. Everything is done autonomously as the AI both tests the original query and learns and modifies in between. The more data it gathers from the user experiences, the more it can modify the landing page, suggesting segmented information to various cohorts to optimize effectiveness.
Therefore, A/B testing adjusted is not only no longer needed after the fact, but pages are forever optimized. Furthermore, there's no longer a need for marketers to play the guessing game. No longer are there complicated spreadsheets that need analysis to identify trends; AI will call them all out. A/B testing reduces the time of trying to improve conversion rates and makes the action far more effective and intelligent. Marketers can feel comfortable that even in their absence, the landing page will be optimized in real time.
Ultimately, AI A/B testing is a game-changing solution to landing page optimization. Greater optimization via simultaneous testing, immediate adjustments, and continuous result assessment allows a marketing campaign to be perpetually one step ahead of the target audience's needs, fostering a seamless experience that's quick and efficient.
Predicting and Personalizing Based on User Behavior
AI doesn't just use A/B testing to optimize landing pages it studies behavioral trends and personalizes adjustments from that angle. For example, if a user visited a certain website before and exhibited certain behavior, AI tracks this and deduces what imagery or copy will work best for this user or similarly-sized groups of users in the future. If a user consistently views the page about pillows and blankets but very rarely on sheets, AI might adjust the imagery on the landing page to show more pillows and blankets and tone down the call to action for sheets. The AI can adjust copy, images, and more based on previous visits.
This personalization above and beyond an A/B testing standard of switching elements to see what works renders AI-driven engagement more successful because the more times a landing page is adjusted for one user over time, the more it can understand similar users. Therefore, each interaction makes the following interactions better and, in the moment, creates a more engaging experience. Users will stay on the page longer and convert better when it seems what they're looking at is tailored directly to them.
The Future of Landing Page Optimization
The future of A/B testing and landing page optimization lies with AI. As this technology advances, it'll be even better positioned to render a high-converting personalized landing page without human involvement. In a few short years, AI could become sophisticated enough to process and evaluate deeper, complex interactions across more channels. For example, it could gain information from social media and evaluate how an email may have impacted someone opening and clicking through the landing page and even informed physical interactions to create a comprehensive experience.
Furthermore, as AI becomes less costly and easier to implement, small businesses and budget-conscious marketers will have access to the same automated A/B testing and adjustments that enterprise level would have; what was once high-level conversion rate optimization is open to all. AI can allow marketers to work on the overarching strategy and creative execution while AI manages implementation and gathers adjustments for better landing pages and campaigns.
Eliminating the Guesswork from A/B Testing
Since digital marketing began, A/B testing has been a popular way for teams to execute enhancements and engagement with landing pages, ads, and websites to drive conversions. However, A/B testing is a standard process that requires a significant amount of educated guessing. Marketers get a suggestion for change based on a campaign that worked before, the trending best practices, or ideas about how a landing page is used by an average user. Yet while educated guesses work based on macro data, human bias relies on the effectiveness of guessing what works for one small segment or micro campaign doesn't necessarily translate across the board.
Thus, the problems with functioning this way are that educated guesses fail more often than not based on segments and conditions. For example, an educated guess based on a macro audience does not always help a micro, niche audience. An uninspired call to action (CTA) may turn someone off in one audience but attract attention in another. Therefore, A/B testing becomes ineffective when audiences do not align with educated guesses. What ends up happening is further time spent testing to determine which educated guess will ultimately become the correct course of action.
A/B testing with AI integration alters this experience because it's no longer guesswork. Guessing comes from prior conclusions met or mere guessing without present-day knowledge. But AI programs have access to real-time data of who is currently online and in what capacity, tracking their behaviors, clicks, and engagement. AI can instantly infer who a given feature works for and why whether it's because of their history on the page, their geo-location, or even their sex. AI will pick up on the slightest variances in intent that the human eye might miss in an A/B testing experiment.
AI A/B testing does not necessitate the editor to choose a path or set a guideline tested on limited offerings, for it can always assess results and gauge intuitive responses by the data compiled. For example, should a specific CTA be performing better with people from a specific town, AI can edit the page to reflect that change in real-time. Should people who are visiting your site for the first time gravitate more to a certain headline, AI will edit it to reflect their feedback. In this way, landing page experiences are always optimized for the audience in question instead of for faulty conclusions from yesterday's visitors.
Therefore, this seamless shift from A/B testing based on assumption to live data-driven improvement increases not only the accuracy of landing page adjustments but also their effectiveness. AI understands based on data received in real-time instead of waiting for an A/B test to render its results. Where human marketers may only be able to test a limited number of options, AI operates in the background, effortlessly 24/7, constantly improving landing pages and similarly rendering users' experiences more personalized for greater returns with significantly less effort.
Furthermore, AI-enhanced A/B testing increases the efficiency of the entire process. For instance, just reaching enough data from a static version can take weeks or months on the traditional side before marketers can proceed with an informed guess. With AI, the algorithm can assess and adjust sooner, making instantaneous alterations that enhance effectiveness in the present. Marketers are no longer stuck to long testing durations and outdated findings afterward; AI enables faster, better-trained findings to champion effectiveness in the now.
Ultimately, through AI-driven A/B testing, marketers are able to move forward with certainty and confidence in what once was uncertain territory. For now, with AI eliminating the guessing game for simple optimization, marketers can rest assured that whatever changes are made will impact performance in some quantifiable fashion and most likely for the better. Conversion rates rise because the experience becomes more fluid due to AI facilitating all the moving parts that a marketer doesn't have time to manipulate. Therefore, where AI can take care of low-level decisions, marketers can focus on the overall goal and allow AI to power their newly found optimization.